Scientific program
- Overview
- Online Program
- Panel Debate
- Short Abstract Volume
- Key Topics
- Contributed Sessions
- Methods
- Areas of Applications
Overview
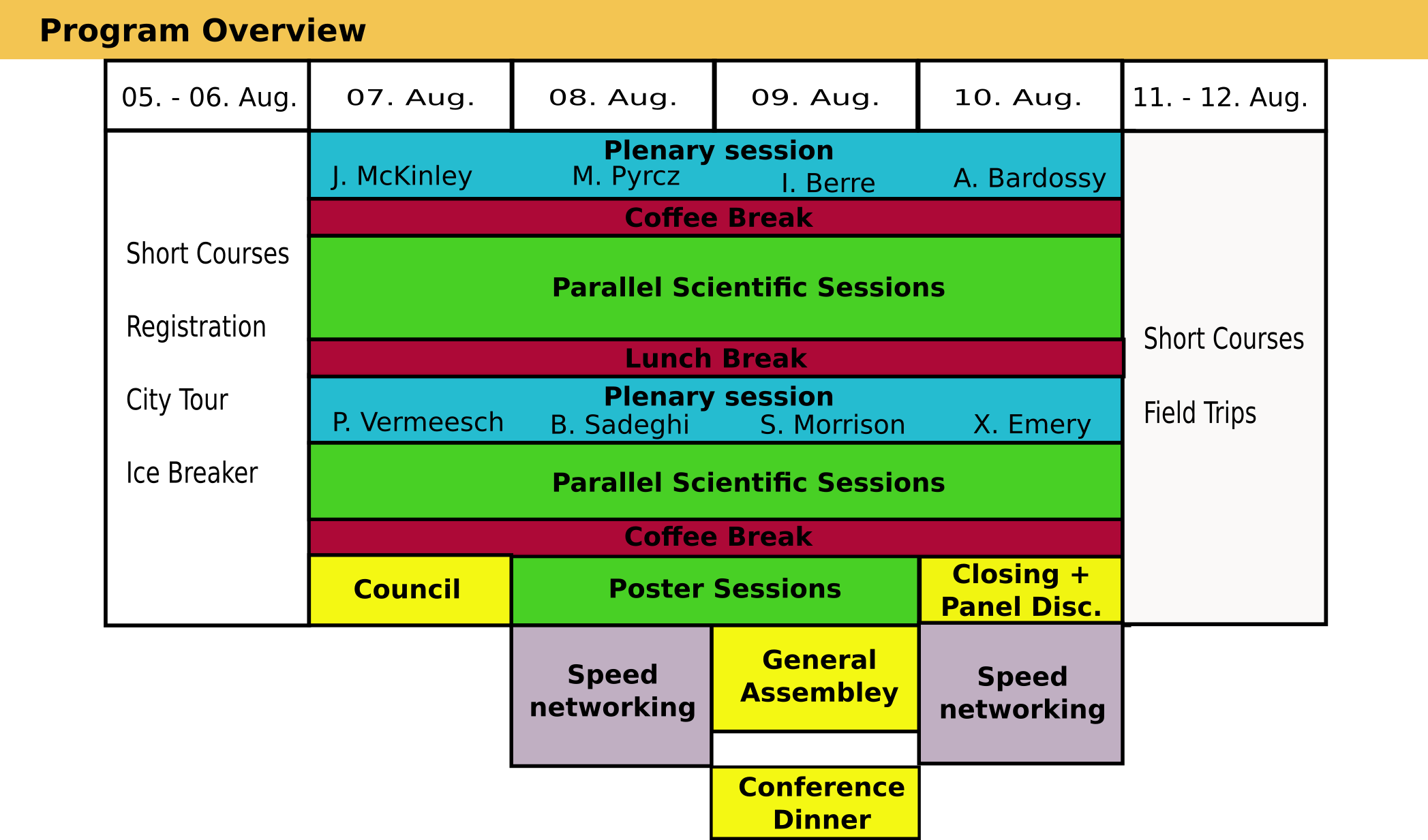
Program
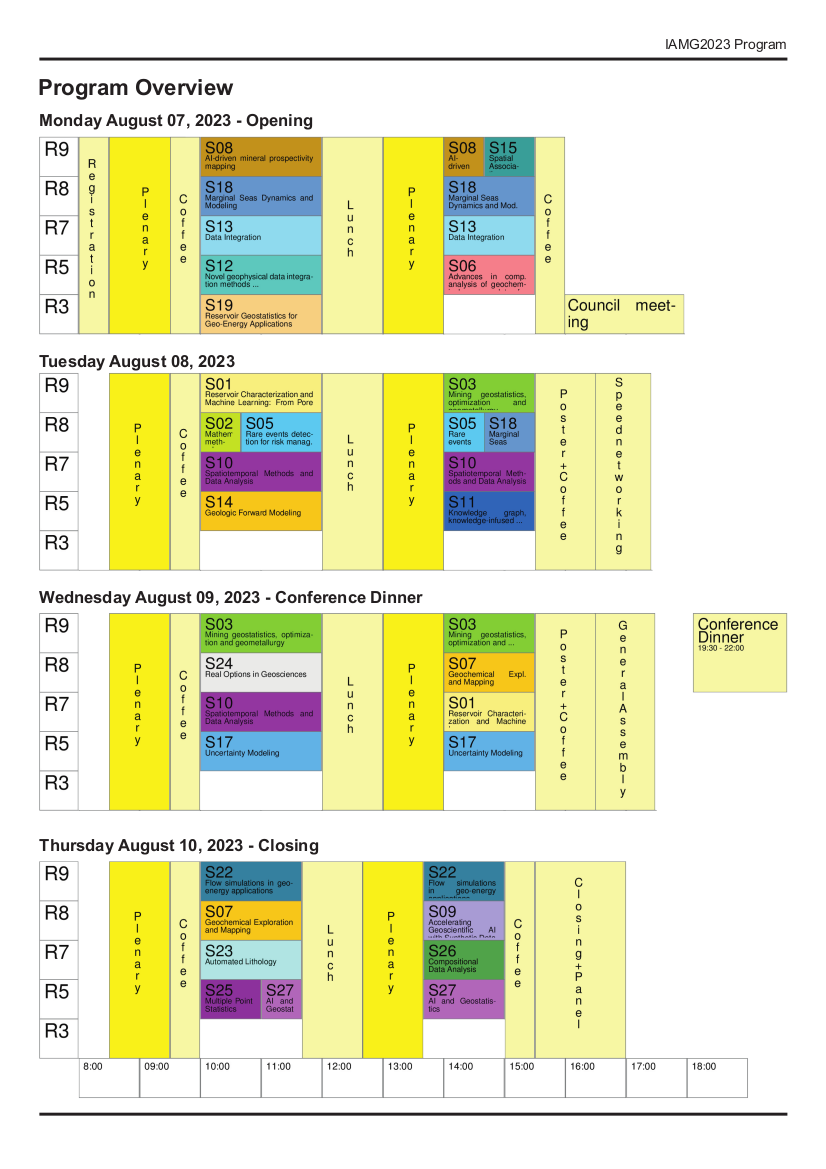
The conference welcomes contributions to all areas of mathematical geosciences and geoinformatics. Contributions can either be submitted to
- contributed focus sessions,
organized around current hot topics by conveners from these subject areas,
- or without a preselected session to general sessions
organized for clusters of topically related contributions by the sciencific committee.
Panel debate:
How can the integration of AI and machine learning approaches with geostatistics be achieved without compromising the rigorous statistical foundations of traditional geostatistical methods?
August 10th, Thursday 4:00-5:00 pm - Closing Ceremony
The emergence of big data, AI, and machine learning has brought about new opportunities and challenges for earth modeling. While traditional geostatistical approaches may not be suitable for handling large and complex datasets, new hybrid approaches that are scalable, flexible, and interpretable are needed to help overcome these limitations. The panel will address how the integration of AI and machine learning with geostatistics can lead to more accurate, reliable, and scalable geo-scientific models if pitfalls like uncertainty bias, insufficient or low-quality labeled data, and lack of interpretability can be mitigated.
The panelists will further debate how the mathematical geoscientist can contribute and enhance insight into the integration of AI and ML with geostatistics?
A Special Issue of Mathematical Geosciences will be dedicated to how AI and machine learning approaches can be integrated into geostatistics without compromising the rigorous statistical foundations of traditional geostatistical methods. This issue will comprise follow-up papers and a transcription of the panel debate. A call for papers for the special issue will be announced at the IAMG23 August 2023.
Moderator:
Richard Sinding-Larsen, Professor Emeritus, Norwegian University of Science and Technology, Trondheim.
Panelist:
- Jennifer McKinley, Professor of Geology and Mathematical Geoscience, Queen's University Belfast, UK
- Shaunna M Morrison Research Scientist, Earth and Planets Laboratory, Carnegie Institution for Science, USA
- Lingchen Zhu, Research Scientist, Schlumberger-Doll Research Center, Massachusetts, USA
- Thomas Mejer Hansen, Associate Professor, Department of Geoscience, Aarhus University, Denmark
- Odd Kolbjørnsen, Advisor in Data Science at AkerBP and Associate Professor at the University of Oslo, Norway
- Gerald van den Boogaart, Professor of Applied Stochastic at Institute of Stochastics, TU Bergakademie Freiberg, Germany
Short Abstract Volume
Can be downloaded here.
Contributed Sessions

Reservoir Characterization and Machine Learning: From Pore to Field Scale
Suihong Song, Yuqi Wu, Mingliang Liu, Senyou AnCharacterization and modeling of subsurface reservoirs at different scales (i.e., from pore scale to field scale) is of great significance to understand inner geological structures, reveal transport mechanisms of various phenomena, predict physical properties, and forecast fluid flow in the future. In recent years, machine learning has exhibited great potential to improve the characterization and modeling accuracy of subsurface reservoirs. In the session, we welcome innovative machine learning approaches and exciting applications related to reservoir characterization and modeling of various scales. The key topics include, but are not limited to: (1) Characterization and modeling of reservoirs at the core or field scale based on traditional geostatistics; (2) Machine learning methods for geo-modeling of macro-scale reservoirs and reconstruction of micro-scale digital cores; (3) Machine learning methods for the upscaling of reservoirs from pore to field scale; (4) Machine learning methods related to the digital rock images (e.g., denoising, segmentation, and enhancement); (5) Data-driven or physics-informed methods for geophysical/flow simulation and inverse problems; (6) Application of the above methods for various fields (e.g., petroleum, mine, groundwater, CO2 storage).Mathematical methods and approaches for monitoring and predicting ground movements
Jan Blachowski, Jörg Benndorf, Steinar L. EllefmoThe session focuses on topics related to theoretical and applied studies of phenomena related to the effect of mining and post-mining on their surroundings. Such as: - application of GIS, spatial statistics, machine learning and deep learning for modelling of spatial relationships in mining areas, - applied earth observations for monitoring of mining activity, - multi-source data fusion for analysis and modelling of mining areas, - assessment of mining activities to mitigate their environmental impacts, - case studies of innovative methods and applications.Mining geostatistics, optimization and geometallurgy
K. Gerald van den Boogaart, Julian Ortiz, Raimon Tolosana Delgado, Jörg BenndorfThe sessions aims to bring together all aspects of mining-relevant mathematical methods, along the whole mining cycle from exploration targeting to mine closure and on all scales from microstructure characterization to the long term mine planning and multi mine site operation planning. Important areas are: potential mapping, microstructural modelling and observation, geostatistics of geometallurgical variables, process modelling, high order geostatistical simulation for geometallurgical variables, structural modelling with uncertainty, stochastic mine planning, real time mining updating, and predictive process optimization. Contributions from all fields of application or development of geomathematical methods for mining are welcome.Insights into Sustainable Water Resources Management using Integrated Geospatial and Geophysical techniques
SUJAY BANDYOPADHYAY, Tapas AcharyaWater resources play a significant role in sustaining life for all living things. The ever-growing population and fast urbanization are leading to over-utilization of water resources, exerting pressure on these commonalities, which are on the brink of collapse. Essentially, rapid and unplanned urbanization significantly damage groundwater and surface water resources owing to the lack of proper initiative. Not only the high inhabitant expansion, but the world climate has also been changing more rapidly in recent years, causing extra difficulty in appropriate water management, including storage plans. Therefore, managing water resources worldwide is a major challenge in the nexus of population growth, climate, and land use change. However, adopting integrative approaches plays a crucial role in managing the country's available water resources towards evolving a sustainable management plan for proper conservation and utilization of water resources. The proposed session will provide a forum for showcasing novel and emerging research to address these pressing global water challenges of the 21st century. The session intends to bring together a variety of scientific fields focused on surface and groundwater resources management using a holistic approach that includes geological, geospatial and geophysical techniques. Presentations may cover a wide range of approaches, including analytical and numerical modelling using geospatial data, machine learning and multi-criteria decision making approaches and exciting geophysical applications related to water resources management of various scales. We particularly encourage submissions that will make a significant contribution and provide valuable data for the sake of integrated sustainable water resources management.Rare events detection for risk management in geoengineering
Alla Sapronova, Thomas MarcherProposed title: Detecting rare events with data-driven modeling. Session description: The session will address the problem of rare event detection: with a vast amount of collected observations, the events having a significant impact and long-lasting consequences are often the most uncommon in frequency. We propose to discuss tools, methods, and current best practices for rare event detection in geosciences, with a particular focus on risk management and its application in geoengineering. The session will present new proposals and findings from the scientific community that include but are not limited to data preprocessing, feature engineering, rebalancing, application of statistical or machine learning methods, and demonstrations of progress in rare event detection that can promote a proactive approach in risk management. This section will cover: 1. overviews of methods and recommended workflows for handling data sparsity and uncertainty; 2. reviews of new findings and techniques for rare events’ detection and recommendations on data preprocessing methods that help to ensure both data quality and novelty in patterns; 3. reports on how various methods for handling data imbalance affect the accuracy of forecasting data-driven models; 4. approaches used for detecting perceptions and biases in data labeling and interpretations; 5. demonstrations of methods and tools successfully employed for risk modeling and rare event detection.Advances in computational analysis of geochemical survey data for geochemical anomaly detection
Behnam Sadeghi, Peter Atkinson, Jennifer McKinley, Mark CooperComputational analysis has been developed in various fields of geosciences, especially mineral exploration and environmental studies. Such analyses, including data exploration and visualization, geostatistics, uncertainty modeling, and machine learning (ML) methods, integrated with remote sensing, and geochemical data, can provide greater identification and understanding of potential geochemical anomalies or contaminations on, or under, the ground. In this session, we welcome abstracts related to geochemical anomaly detection and characterization with remote (and, thus, a large number) geochemical surveys covering the following topics: (1) spatial resolution choice, sampling design, image analysis, deep learning, higher-order representations, spatial object representations, (2) mathematical/statistical anomaly classification methods to define geochemical anomalies in 2D to 5D such as multifractal modeling, ML, simulations, and spatial uncertainty evaluations, and (3) any relevant applicable data analysis, particularly geospatial and compositional data analysis (CoDa). The main focus of this session is to integrate the mentioned methods to come up with highly efficient and reliable representative models and exploration decisions with the lowest possible uncertainties and risks.Practical Aspects and Applied Studies in Geochemical Exploration and Mapping with Compositional Logratio Techniques
Jennifer McKinley, Karel HronThis session will offer a practical forum of discussion for people concerned with the statistical treatment, modelling and interpolation of compositional data in geochemical applications, particularly focused on geochemical exploration and mapping. The session will invite papers related to the problems of geochemical mapping, and the compositional topics. Geochemical survey data have both compositional and spatial character. Thus, there are two spaces for these data; the geochemical and the geospatial domains. The multivariate relationships of the elements typically reveal patterns associated with processes determined by mineralogy, weathering, comminution and mass transport. Typically, these processes display a continuous spatial character in both 2 and 3 dimensions and time. Many methods are available for process discovery such as principal components or minimum/maximum autocorrelation factor analysis. The evaluation of geochemical data can be carried out using the approach of process discovery and process validation. In the context of Process Discovery, different methods use different measures of association. Some multi-element associations are common amongst all of the metrics, whilst other measures are unique to the metric. These metrics use both linear and non-linear mappings to define the associations. Typically, the approach is unsupervised with the intent to define multi-element associations that reflect mineralogy associated with rock types. When the procedure of process discovery identifies patterns that represent geologic phenomena, these patterns can be tested using classification methods and is termed process validation. Methods such as discriminant analysis operate in linear and non-linear modes to carry out prediction. Neural networks, logistic regression, random forests, support vector machines use various measures of association for classification using heuristics such as decision trees and multivariate measures of distance. This approach is usually described as a supervised way of testing the classes defined through process discovery or by pre-defined categorical information assigned with each geochemical composition. This session invites contributions where multivariate geoscience data (geochemistry, geophysics, geodetic, geologic) can be processed within a compositional framework that includes an evaluation of the data, or results of processing the data, in a geospatial context.AI-driven mineral prospectivity mapping
Renguang Zuo, Emmanuel John M. Carranza, Yihui XiongMineral prospectivity mapping as a computer-based approach to delineate targeted areas for a specific type of mineral deposits has changed from being knowledge driven to today’s AI-driven. There are increasing applications of artificial intelligence (AI) in mineral exploration. The session welcomes the following submissions: (1) novel AI algorithms and application of AI for processing geological, geochemical, geophysical, remote sensing and drilling data; (2) novel AI algorithms and application of AI for identifying and integrating prospecting features in support of mineral exploration.Accelerating Geoscientific AI with Synthetic Data
Peter Tilke, Hakan BasarirData is crucial for implementing, training and applying AIML solutions for subsurface interpretation. Synthetic data can generate labeled realistic data efficiently and at scale for AIML applications. It is non-identifiable so privacy and data security concerns don't apply. Can be used to train and test AIML models, for exploratory data analysis, to validate assumptions, demonstrate results that can be obtained with AIML models, and reject models producing poor results without the cost of acquiring and incorporating real data. Can be used for transfer learning. In this session we would like to share experiences with generating synthetic geoscientific data (e.g., forward modeling) for the purpose of accelerating AIML workflows. Building a synthetic data library of geologic analogs represents a level of complexity which involves creating, managing and accessing vasts amounts of data efficiently for a variety of use cases.Spatiotemporal Methods and Data Analysis
Dionysios Christopoulos, Sandra De Iaco, Emmanouil VarouchakisThe development of spatiotemporal models is at the forefront of current research in Geostatistics and Machine Learning. Currently there is a need for models that can accurately capture the complex patterns observed in various dynamic processes and to reliably estimate uncertainties. Key modeling challenges for the analysis of modern spatiotemporal data involve the development of models suitable for large data sizes, heterogeneous data, multivariate dependence, multiple correlation scales and non-Gaussian probability distribution. This session seeks contributions that will advance spatiotemporal Geostatistics by proposing novel concepts and methodologies, computational algorithms, and innovative applications or studies that focus on spatiotemporal data analysis. An indicative but non-exhaustive list of topics includes the development of novel space-time covariance functions (e.g., non-separable models, models on the sphere and manifolds, multivariate dependence, complex-valued models), covariance-free approaches (e.g., models based on stochastic partial differential equations and explicit precision operators), innovative simulation methods, computational advances for big space-time datasets. The session also invites contributions that focus on applications involving interesting and/or challenging spatiotemporal datasets, as well as approaches for non-Gaussian space-time data and multiscale models. Contributions that combine Geostatistics with current developments in Applied Mathematics (e.g., uncertainty quantification and multifidelity frameworks), and Machine Learning (e.g., hierarchical models, sparse and multiscale Gaussian process regression) are also welcome.Knowledge graph, knowledge-infused machine learning, and neuro-symbolic AI in geosciences
Xiaogang Ma, Anirudh Prabhu, Xiang Que, Chengbin WangKnowledge graph, as an umbrella topic for works on ontologies, controlled vocabularies, and knowledge engineering, has received significant implementation in various disciplines of geoscience. Very recently, in the field of artificial intelligence (AI) and data science, the topics of knowledge-infused machine learning and neuro-symbolic AI arise as cutting-edge areas, which aim to combine the rule and logic-based approaches with the state-of-art machine learning methods. Knowledge graph is a representative method in the rule and logic-based approaches, but many previous studies were in the domain of data curation and integration, and there was limited work on infusing knowledge graphs with computational processes such as spatial statistics and machine learning. The new focus on knowledge-infused machine learning and neuro-symbolic AI provides a venue for researchers to review and reflect the role of knowledge graph in data-intensive geoscience studies. This session welcomes submissions on perspectives, methods, and case studies of those topics mentioned above.Novel geophysical data integration methods to assess natural resources
Katherine Silversides, Guillaume Pirot, Jeremie GiraudThe session focuses on applying novel methods to geophysical data integration for the exploration and characterization of natural resources including mineral deposits, water, hydrogen and geothermal energy. Proposed techniques may include multi-disciplinary integration approaches and applications of machine learning to geophysical integration problems.Data Integration
Jo Eidsvik, Thomas Mejer Hansen, Klaus MosegaardMany geoscientific challenges require the integration of information from diverse sources, such as geology, geophysics, geochemistry, and hydrology. This calls for methods that allow a) quantifying information, and b) integration of information. Once all information has been combined, it needs to be made available to the end-users, e.g. the decision makers, in a way that allows taking an informed decision based on all available information. For this session, we invite contributions that deal with any of these aspects related to data integration in geoscience, with the goal of allowing decision-makers to take an informed decision. These contributions can for example be based on inverse problem theory, geostatistics and machine learning.Geologic Forward Modeling
Daniel Tetzlaff, Scott Bowman, Sergio Courtade, Gerard MassonnatMethods, algorithms, and practical applications of models that simulate physical geologic processes through time, including sedimentation, stratigraphy, compaction, tectonics, diagenesis, heat flow, and hydrocarbon maturation and migration. Also, hybrid techniques that use geostatistics, machine learning, and artificial intelligence in combination with forward modeling to improve data matching and enhance predictability away from data.Spatial Association
Yongze Song, Qiuming ChengThis session invites researchers to present the latest methods of spatial association and their implementations. Effective characterisation of the spatial association is the basis for spatial factors exploration, spatial prediction, and geographical decision-making. Currently, models of spatial dependence, heterogeneity, singularity, similarity, and other structures of spatial data are the mainstream to describe spatial association. Recent studies demonstrate that geospatial artificial intelligence also provides accurate and effective solutions for spatial association analysis. This session welcomes submissions from both methodology and application studies about the spatial association.Uncertainty Modeling
Florian Wellmann, Clare BondUncertainties are prevalent in many geoscientific studies: from geostatistics and 3-D geological modelling to coupled process simulations. In this session, we invite contributions covering aspects of uncertainty estimation, modelling and visualisation in geosciences. A special focus will be on (1) methods to estimate uncertainties in data sets, expert elicitation and the analysis of bias (2) approaches to propagate uncertainties through modelling and simulation, including surrogate modelling, probabilistic programming and machine learning approaches, and (3) the quantification and visualisation of uncertainties in temporal and spatial domains. We encourage contributions both addressing theoretical and methodological challenges, as well as applications to geoscientific problems.Marginal Seas Dynamics and Modeling
Jan Harff, Joanna Joanna Dudzińska-Nowak, Wenyan ZhangMarginal seas form a belt of transition between land and ocean. This chain, crossing all types of climate zones, having formed under various tectonic and geological conditions plays a crucial role in providing people with habitat, food, trade ways, and facilitated socio-economic networking. However, marginal seas are increasingly threatened by rising sea-level, floods, storms, tsunamis, coastal erosion and environmental hazards that endanger livelihoods. These threats have become even more visible in recent times in the face of climate change and anthropogenic impact including industrialization on the natural environment. The DDE Marginal Seas Task Group co-organizing the session is inviting geoscientists, modelers and IT scientists to discuss the opportunities to mitigate the threats by the application of advanced data acquisition such as monitoring concepts, big data analyses, numerical modelling, AI and Machine Learning for sustainable development of the coastal and marine realm of marginal seas. These models allow the reconstruction of the geological past as well as the generation of future scenarios that can be used for effective planning of sustainable development. The dynamics of sea level change, morphodynamic processes, anthropogenic pressure, model design and standardized solutions for sustainable development will play a principle role at the session. Networking of the international scientific teams will be explicitly supported.Reservoir Geostatistics for Geo-Energy Applications
Juliana Leung, Sanjay Srinivasan, Baehyun MinGeostatistics has been widely applied for modelling subsurface flow systems encountered in many geo-energy applications, including but not limited to oil and gas extraction, geological storage of CO2, and geothermal reservoir development. Traditional workflow entails generating multiple reservoir models conditioned to data from diverse scales and sources, subjecting these models to flow or transport modelling, and updating the models with new dynamic data. We are soliciting abstracts that showcase recent advancements in the characterization of complex reservoirs, integration of multifaceted data from diverse sources recognizing the scale and precision of such data, upscaling of flow or transport properties, and coupling machine learning with traditional modelling workflows for uncertainty quantification. Interesting case studies, as well as novel theoretical and computational developments, are welcome.Flow simulations in geo-energy applications and gas storage
Francesca Watson, Elyes AhmedNumerical flow simulations are crucial for all kinds of geo-energy and geological gas storage applications. This is a broad area with aspects relating to spatial discretisation of the flow equations over complicated domains, numerical solution of potentially large systems of equations, models with high levels of heterogeneity and limited data availability, to name but a few. In this session we invite speakers to present recent advances on topics regarding flow simulations in the subsurface. Contributions are welcome in areas such as effective solution methods, data-driven modelling, flow simulations for various applications e.g. geothermal, fractured reservoirs, CO2 storage, as well as anything else relevant to the field.Automated Lithology
Kurt Aasly, Eric PirardAutomated characterization of minerals and rocks is of major importance for the future development of numerical geology. Exemplified with the mining industry, where this is known in core-scanning and automated mineralogy. The vast amount of data created from various analytical methods, creates sets of «big data» that, if properly analyzed, may be significant to improve the understanding of mineral deposits, their exploration, and their downstream processing. Recent technologies, based on both 2D and 3D imaging systems, in combination with the large number of data from geochemical analyses provides sets of big data that should be efficiently analyzed together using AI and ML methods. This session welcomes submissions that offer contributions on the approach for analyses of such big datasets and case studies.Real Options in Geosciences
Verena Hagspiel, Milan Stanko, Luis Martinez TipeEnergy and mining sectors play a critical role in the modern economy. These are capital intensive sectors tied to products that are commonly traded on financial exchange. Another characteristic is that projects in these sectors are prone to large private risk related to resources as well as available technology. Therefore, advanced valuation techniques and forecasting can significantly improve economic aspects associated with the development and operation of projects. Real options analysis allows to quantify the value of managerial flexibility inherent in potential projects. Managerial flexibility stems from the decision maker’s ability to react on uncertainty as it unfolds over time. Project development related to mining or energy can in many cases be split into a sequential problem with multiple decisions over time. Using the knowledge gained between stages, the management can optimize future decisions. This allows to some extend mitigate downside risk and exploit the upside potential of uncertainty. Real options valuation provides us with a tool to quantify the value of such flexibility and identify the most profitable decision strategies. In this session we aim to bring together experts as well as newcomers to the topic that are interested to learn about practical applications of real option valuation to decision problems in energy and mining sectors.Multiple Point Statistics
Jacob Skauvold, Håkon TjelmelandMultiple Point Statistics (MPS) has developed tremendously during the last 20 years - the main idea being to move away from the traditional (Gaussian) two-point dependency models. Based on extensive training data, MPS can provide realistic discrete (or continuous) realizations of geomodels for facies or other attributes. Over the years more complex MPS models have been developed, and one can say that this has sparked interests in using recent machine-learning approaches such as Generative Adversarial Networks which are currently quite popular in the field.Compositional Data Analysis
Juan Jose Egozcue, Vera Pawlowsky-GlahnCompositional Data Analysis (CoDA) can be applied advantageously in many fields. However, CoDA still needs methodological improvement. This session is focused on such applications in geosciences and includes methodological contributions.AI and Geostatistics
Jo EidsvikThere is currently tremendous progress in the field of artificial intelligence (AI) providing optimized decisions, plans or learning systems via sophisticated computer programs. In this session authors study how the development in AI links to geostatistical models and methods. Some presentations combine AI and geostatistics for various geoscience applications, while other outline AI methods that can potentially overcome challenges with more traditional geostatistical approaches.
Methods
Areas of Application
Key Topics
- Mining geostatistics and geometallurgy
- Meshing
- Machine learning
- Non-linear regression
- Optimization
- Petroleum geostatistics
- Tectonics
- Uncertainty modelling
- Geochemistry
- Flow in porous media
- Resource potential estimation and prospectivity mapping
- CODA
- Geodynamics
- Analysis of spatio-temporal data
- 3D (geometric) modelling
- Computational petrology
- Geophysics and inversion
- Geologic forward modeling
IAMG2023 sponsored by:
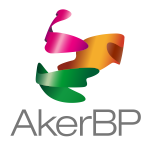
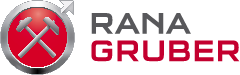
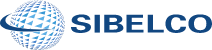